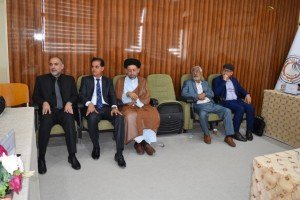
A master's thesis in the College of Engineering at the University of Basrah discussed (recognizing human gestures based on the use of a WiFi signal) The thesis presented by the student, Sukaina Kazem Jawad, dealt with the realization of the human gesture recognition system based on the CSI value provided by WiFi devices. The system included several different stages and methods, including pre-processing, feature extraction, and classification, to improve discrimination accuracy. Four strategies are presented to increase the discrimination accuracy of the system. In the first strategy, new features in the time domain TD and time domain TFD that were manually extracted from the CSI amplitudes were proposed. In the second strategy, four deep convolutional neural network models are proposed for extraction purposes Features and classification using the concept of transfer learning. The modification of the models was done by using the concept of transfer learning TL from some layers of the pre-trained models to “extract the features in the modified models”, In the third strategy, four hybrid models are proposed that combine these models with deep convolutional neural network models The fourth strategy focused on building a 1D-CNN from scratch to distinguish the types of movements. The inputs to this model are the CWT continuous wave transform features amplitude of CSI signals for 16 types of motions. The thesis aims to distinguish human gestures based on the use of the WiFi signal The thesis concluded that comparison with previous studies shows the effectiveness and strength of the proposed methods in determining human gestures