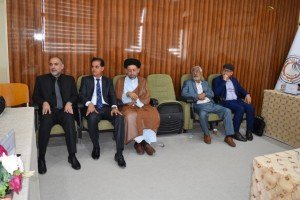
The doctoral thesis of student Haider Suhail Najm was discussed at the University of Basra, College of Engineering, Department of Mechanical Engineering, which discusses the early and cross-domain detection of vibration of bearings with rolling elements using artificial intelligence techniques.
In this work, fault diagnosis intelligence techniques are developed to address two fundamental challenges in vibration analysis in ball bearings. First, these techniques must be able to detect and classify faults in the early stages of their development, as vibrations during this stage typically have small amplitudes, making them difficult to capture.
Second, the developed methods should be applicable to fault diagnosis in a variety of ball bearing types, rotational speeds, and loading conditions.
The researcher concluded that the results of the cross-domain analysis using the classifier (FD-ANN) with the highest accuracy reached an average accuracy of 98.33% for the (NU 205) bearing, and an average accuracy of 97.3% for the defective bearing group, with an average success rate of 99.81% significantly achieved for the bearing. (Koyo 1205) under loading conditions equal to (1.325 kg)