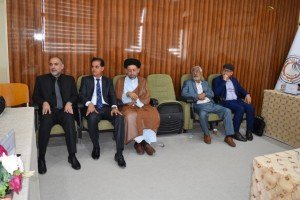
Graduates of the Department of Petroleum Engineering at the College of Engineering, University of Basra participated in the International Petroleum Engineering Conference entitled “Unconventional Resources Technology Conference,” University of Basra - College of Engineering, and Schlumberger SLB.
Spotfire Company - USA includes search A two-stage, data-driven, end-to-end framework utilizing machine learning is developed, evaluated, and presented to optimize the rate of penetration (ROP) in S-shaped wells. This framework employs prior knowledge from existing wells in Southern Iraq to automatically identify optimum values for controllable parameters. The framework places greater emphasis on the similarity between wells using Dynamic Time Warping (DTW) techniques to prioritize knowledge usage from highly similar wells. Additionally, it adapts to new field data and domain experts' recommendations. The framework's results were compared with those of existing wells, and the percentage of improvement was presented. The paper focused on modeling the ROP following a data driven approach. 4 models were used to acquire the results, Support Vector Machines (SVM), Artificial Neural Networks (ANN), Random Forest, and XGboost. These models where used to correlate the ROP with 15 other independent parameters like revolutions per minute (RPM), weight on bit (WOB) in the prediction models. The accuracy of these models then evaluated based on the correlation coefficient. The algorithm with the highest correlation coefficient is stored to be used as an objective function in the optimization algorithm. The WOB, RPM, SPP, and Flow Rate were adjusted to their optimum value with the optimization algorithm which was Particle Swarm Optimization (PSO). The data set consist of 4 wells, and 8032 data points form the same cluster of wells. The data is split into 0.75 for training and 0.25 for testing, for the ROP prediction phase or Stage 1. The evaluation results based on the correlation confection for each algorithm were found to be as follows: 0.703 for SVM, 0.915 for ANN, 0.966 for random forest, and 0.992 for XGBoost, and the testing data set evaluation resulted in the following correlation coefficient of 0.728 SVM, 0.828 ANN, 0.929 for Random Forest, and XGboost 0.946. XGBoost was used as the objective function for Stage 2. The ROP improvement for the four wells was as follows, Well#1, 18.13%, Well#2 19.3%, Well#3 18.67 %, Well#4 19.67%.
https://onepetro.org/URTECONF/proceedings-abstract/24URTC/3-24URTC/D031S068R004/546942